Stay Ahead in Tech: Top Machine Learning News Updates
Buckle up, tech enthusiasts! We're diving headfirst into the thrilling realm of machine learning news and artificial intelligence updates that are shaping our futur
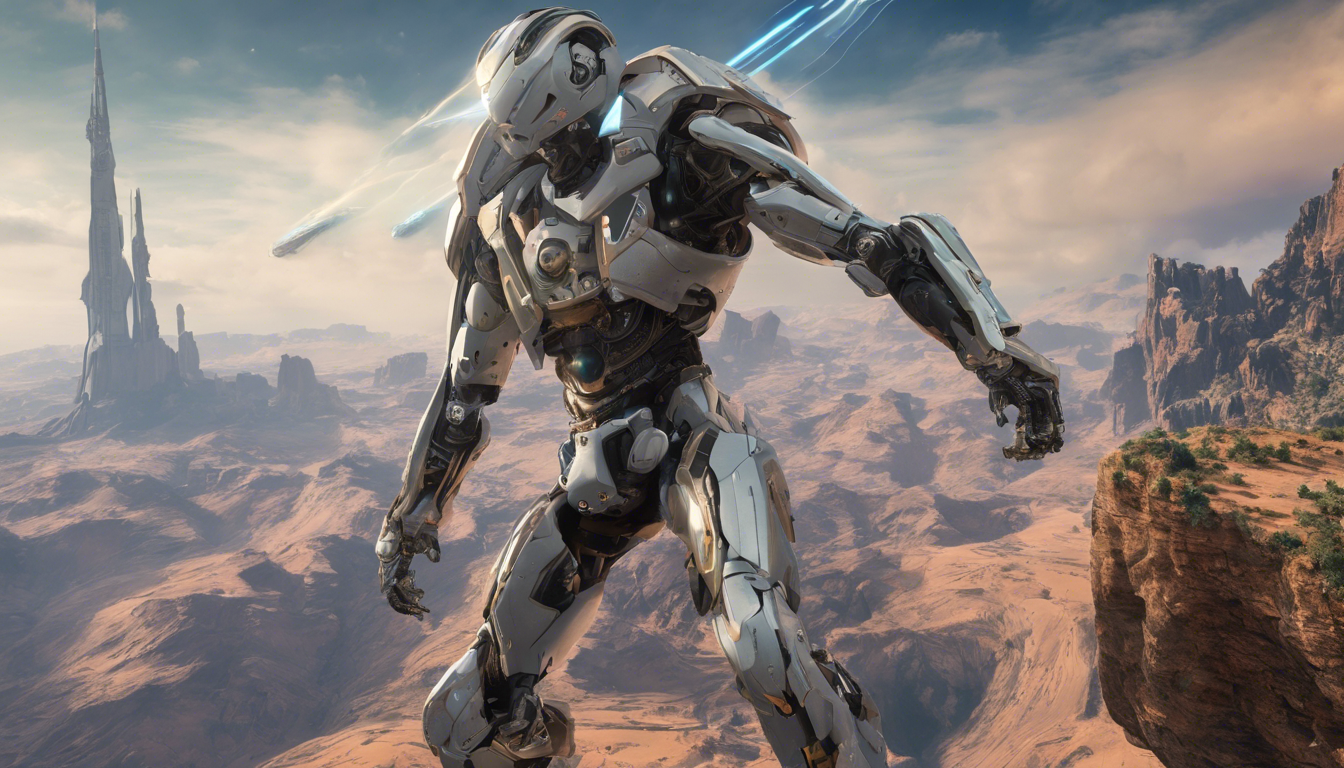
Buckle up, tech enthusiasts! We're diving headfirst into the thrilling realm of machine learning news and artificial intelligence updates that are shaping our future 1. From game-changing breakthroughs to mind-blowing applications, get ready to be amazed by the latest advancements in this ever-evolving field 1.
In this eye-opening article, we'll unravel the top machine learning trends for 2023, including augmented analytics, edge computing, generative models, and the quest for explainable AI 1. Brace yourselves as we explore groundbreaking applications like image recognition, natural language processing, fraud detection, and disease diagnosis that are revolutionizing industries across the board 1.
AI Breakthroughs and Innovations
Advancements Across Multiple Domains
Machine learning is making rapid strides across various fields, including computer vision, personalization, internet search, chatbots, deep learning, and transportation 2. The rise of Explainable AI (XAI) is expected, which aims to make machine learning models more transparent and understandable 3. Increased use of reinforcement learning is predicted, particularly in areas like robotics, self-driving cars, and game playing 3.
Healthcare and Edge Computing
Continued growth of deep learning and increased adoption of machine learning in healthcare are expected 3. More focus on edge computing for machine learning applications that require real-time processing is anticipated 3.
Generative AI and Personalization
Key AI trends include creative or generative AI, ChatGPT, and AI for personalization, which raise ethical questions and require caution 4. AI models have demonstrated near-human levels of comprehension in understanding context, sarcasm, and nuanced human emotions in text, with significant implications for customer service, content creation, and mental health support systems 5.
Healthcare Applications
Integration of machine learning algorithms has led to more accurate predictive analytics in patient care, enabling early disease detection, treatment personalization, and pandemic prediction 5.
Quantum Machine Learning
The fusion of quantum computing and machine learning has led to the emergence of Quantum Machine Learning (QML), which can solve complex problems much faster than traditional computers, with applications in areas like climate modeling, financial forecasting, and drug discovery 5.
Explainable AI
Significant progress has been made in developing explainable AI algorithms that provide transparency and accountability, especially in critical decision-making sectors like finance, healthcare, and law enforcement 5.
Cybersecurity
AI and machine learning are being used to predict, detect, and respond to cyber threats with unprecedented speed and accuracy, with these systems constantly improving their defense mechanisms to protect sensitive data 5.
Industry Applications
AI-Powered Customer Interactions and Marketing
AI-powered tools and platforms are revolutionizing customer interactions and marketing strategies, enabling precise customer identification, personalized messaging, and real-time campaign optimization 4. Machine learning algorithms can analyze vast amounts of customer data, preferences, and behavior patterns to deliver highly targeted and relevant content, products, and services 4.
Predictive Analytics for Business Optimization
AI-powered predictive analytics can help businesses reduce costs, improve operational efficiency, and enhance decision-making 4. By leveraging historical data and identifying patterns, machine learning models can forecast future trends, optimize supply chains, streamline operations, and mitigate risks 4.
Cloud Computing and Domain-Specific Solutions
Cloud computing and industry cloud platforms are enhancing the accessibility, flexibility, and cost-effectiveness of machine learning 9. Domain-specific ML solutions that address the needs of specific industries are gaining momentum, saving time and requiring fewer iterations to meet the use case 9.
Industry-Specific Applications
- Finance and Banking: Over 90% of top 50 financial institutions use AI and machine learning for fraud detection, focused account holder targeting, loan eligibility prediction, and customer churn prediction 12.
- Healthcare: Machine learning is used for drug discovery and manufacturing, personalized treatment and medication, cancer prognosis and prediction, and genetics and genomics 12.
- Retail: Machine learning powers product recommendations, improved customer service through chatbots, and market basket analysis 12.
- Travel: Machine learning enables dynamic pricing (e.g., Uber's surge pricing model) and sentiment analysis of customer reviews 12.
- Media and Entertainment: Features like face detection, recognition on social media platforms, and personalized content recommendations are driven by machine learning 12.
- Engineering: In mechanical engineering, machine learning is used for signal processing and control, while in electrical engineering, it powers motor control, smart grids, and fault detection 12.
- Agriculture: Crop yield prediction, soil analysis, disease detection, and nitrogen modeling are facilitated by machine learning 12.
Cross-Industry AI Applications
Application | Description |
---|---|
Virtual Assistants | AI-powered assistants like Amazon Alexa, Cortana, Siri, and Google Assistant are used across industries 13. |
Chatbots | AI applications used in customer interaction, marketing, and instant messaging 13. |
Healthcare | Computer-aided disease detection, diagnosis, medical research, drug discovery, and diagnosis of life-threatening diseases 13. |
Entertainment | Personalized movie, song, and video recommendations based on user behavior and preferences 13. |
News Aggregation | Customized news based on reader preferences and fake news detection 13. |
Robotics | Robots that can perform human-like tasks, sense obstacles, and pre-plan their journey in real-time 13. |
Advertising | Optimized user experience, data-driven predictive advertising, real-time bidding, and targeted display advertising 13. |
Autonomous Vehicles | Self-driving cars powered by sensors, cameras, geo-mapping, and sophisticated models to navigate through traffic 13. |
Ethical Considerations
Key Ethical Challenges
As machine learning systems become more prevalent and influential, addressing ethical considerations is crucial to mitigate potential risks and ensure responsible development 2 14 17. Some key ethical challenges include:
- Data Privacy and Security: The use of sensitive personal data in machine learning models raises privacy concerns, such as unauthorized access, data breaches, and lack of transparency and control for individuals over how their data is being used 14 17.
- Algorithmic Bias and Discrimination: Machine learning algorithms can inadvertently discriminate against certain individuals or groups based on biases present in the training data or the algorithm itself 14 15 17. Examples include recruiting tools showing bias against women, facial recognition tools exhibiting bias toward certain ethnicities, and AI systems making racist or discriminatory outputs 15.
- Lack of Transparency and Accountability: The opaque nature of many machine learning models makes it challenging to understand how decisions are made, leading to concerns about transparency and accountability when these decisions directly impact people's lives 14 17.
- Ethical Dilemmas in Decision-Making: AI systems may face complex moral dilemmas in decision-making scenarios, requiring a multidisciplinary approach involving ethicists, policymakers, and other stakeholders to establish ethical frameworks 17.
Mitigating Ethical Risks
To address these ethical challenges, several mitigation strategies have been proposed:
- Establishing AI Governance and Policies: Organizations should develop clear policies and guidelines for responsible AI development, promoting a culture of ethics and accountability 15.
- Promoting Diversity and Inclusiveness: Encouraging diversity in AI development teams can help identify and mitigate potential biases, while also ensuring that the needs and perspectives of different groups are considered 15 16.
- Preparing Balanced Training Data: Carefully curating and balancing training data sets can help reduce biases and ensure that machine learning models are trained on representative and inclusive data 15.
- Enabling Explainable AI: Developing AI systems that can provide understandable and transparent explanations for their decisions can build trust and enable users to comprehend and contest the decisions 9 17.
- Conducting Periodic Assessments: Regular assessments and audits should be performed to identify and address potential biases, ethical issues, or unintended consequences in AI systems 15.
- Adhering to Ethical Principles: Organizations should adopt and operationalize ethical principles for machine learning, such as respect for human rights, fairness and non-discrimination, transparency, and accountability 16.
Addressing ethical considerations in machine learning is an ongoing process that requires collaboration among developers, organizations, policymakers, and society as a whole to ensure that these powerful technologies are developed and deployed responsibly 14 16 17.
Future Trends and Predictions
Exponential Growth and Industry Transformation
The machine learning industry is poised for exponential growth, with projections indicating it will reach nearly $226 billion by 2030, a staggering increase from $19.2 billion in 2022 2. This remarkable growth is expected to drive industries toward higher levels of sophistication, with the global ML market anticipated to expand from $26.03 billion in 2023 to $225.91 billion by 2030 9.
Emerging Trends and Advancements
Several exciting trends are predicted to shape the future of machine learning:
- Quantum Computing: The integration of quantum computing capabilities is expected to revolutionize machine learning, enabling the solving of complex problems much faster than traditional computing methods 2.
- All-Purpose Model Development: Efforts are underway to develop a single, all-purpose model capable of handling diverse tasks, potentially leading to a significant breakthrough in artificial general intelligence (AGI) 2.
- Distributed Machine Learning Portability: Increased focus on developing portable machine learning models that can be seamlessly deployed across various platforms and environments 2.
- No-Code Environments: The rise of no-code environments is anticipated, making machine learning more accessible to non-technical users and democratizing the technology 2.
- Reinforcement Learning Advancements: Continued advancements in reinforcement learning are expected, enabling machines to learn from their experiences and make optimal decisions in complex environments 2.
Human-Machine Collaboration
While machine learning will undoubtedly transform various industries, it is not expected to replace humans entirely. Instead, the future is likely to involve a shift in job requirements, with both humans and machines working collaboratively 2. This symbiotic relationship will leverage the strengths of each, with machines excelling at data processing and pattern recognition, while humans provide critical thinking, creativity, and emotional intelligence.
Talent Demand and MLOps
As the adoption of machine learning accelerates, there is an increasing demand for AI/ML talent, especially in areas like MLOps 8. MLOps, short for Machine Learning Operations, focuses on reliability and efficiency in developing ML solutions, managing the ML systems lifecycle from design to deployment and monitoring 9. This emerging field aims to streamline the development, deployment, and maintenance of machine learning models, ensuring consistent and reliable performance in production environments.
FAQs
Q: What are the best ways to stay informed about AI developments? A: To keep abreast of AI advancements, you can subscribe to the "AI Weekly" newsletter for a weekly roundup of the latest in AI research and news. Additionally, following blogs like "The Gradient" can provide you with accessible and insightful articles on AI topics, authored by experts in the field.
Q: Which sources offer the most comprehensive AI news? A: For comprehensive AI news, consider these sources:
- Analytics Vidhya
- AI Trends
- MIT News
- Wired
- Dataversity
- OpenAI
- Emerj
- Extreme Tech
Q: What are some effective methods to stay updated with machine learning trends? A: To stay updated with machine learning trends, you can subscribe to various newsletters, blogs, podcasts, YouTube channels, and online magazines. Notable examples include AI Trends, MIT Technology Review, DataCamp, and content from Lex Fridman.
Q: What is currently the most sophisticated AI available? A: The question about the most advanced AI currently available is not answered here. However, advancements in AI are ongoing, and various organizations and research labs are continually developing and refining AI technologies.
References
[1] - https://www.datalinknetworks.net/dln_blog/machine-learning-ml-the-latest-trends-developments-and-applications-of-ml
[2] - https://365datascience.com/trending/future-of-machine-learning/
[3] - https://www.linkedin.com/pulse/future-machine-learning-predictions-trends-2023-codersarts
[4] - https://www.forbes.com/sites/forbesagencycouncil/2023/09/11/the-future-of-artificial-intelligence-predictions-and-trends/
[5] - https://www.evonence.com/blog/top-5-breakthroughs-in-ai-and-machine-learning-for-2024
[6] - https://www.technologyreview.com/2024/01/08/1085096/artificial-intelligence-generative-ai-chatgpt-open-ai-breakthrough-technologies/
[7] - https://online-engineering.case.edu/blog/advancements-in-artificial-intelligence-and-machine-learning
[8] - https://www.techtarget.com/searchenterpriseai/tip/9-top-AI-and-machine-learning-trends
[9] - https://mobidev.biz/blog/future-machine-learning-trends-impact-business
[10] - https://www.simplilearn.com/artificial-intelligence-ai-and-machine-learning-trends-article
[11] - https://bootcamp.cvn.columbia.edu/blog/machine-learning-applications/
[12] - https://www.projectpro.io/article/10-awesome-machine-learning-applications-of-today/364
[13] - https://www.simplilearn.com/tutorials/deep-learning-tutorial/deep-learning-applications
[14] - https://towardsdatascience.com/ethical-considerations-in-machine-learning-projects-e17cb283e072
[15] - https://www.isaca.org/resources/isaca-journal/issues/2022/volume-4/bias-and-ethical-concerns-in-machine-learning
[16] - https://www.w3.org/TR/webmachinelearning-ethics/
[17] - https://www.dataversity.net/top-ethical-issues-with-ai-and-machine-learning/